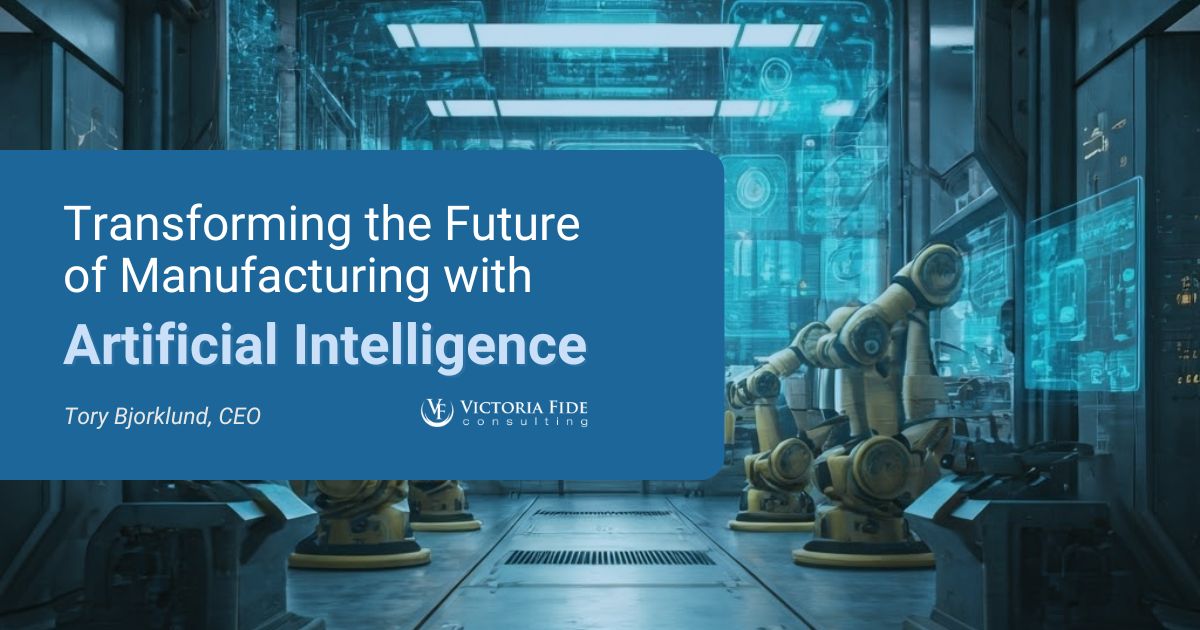
Artificial Intelligence (AI) is continuing to make a splash, but it’s more than a buzzword—it’s here and it’s revolutionizing all types of businesses, including the manufacturing industry. In fact, manufacturing is leading the movement by implementing and leveraging AI to transform the way products are made, delivered and serviced.
For manufacturing executives and CXOs, understanding AI’s potential, challenges, and strategic integration will be a game changer for staying competitive. This blog explores how AI can transform your operations, from highlights of its potential applications to actionable steps for adoption and common risks to consider that will help you prepare to implement AI in your manufacturing business.
Table of Contents
What Are the Different Types of AI?
First, it’s important to acknowledge that not all AI is the same. Whether you have an “AI strategy” or you’re simply using spellcheck, I guarantee all of you are using AI whether you know it or not.
To understand the differences in AI and which tools to select for your manufacturing organization, it’s critical to be able to categorize the AI by what it does.
- Generative AI: Creates new content, such as text (like ChatGPT), images, or music, using machine learning models based on patterns and data sets.
- Predictive AI: Forecasts outcomes based on historical data, such as stock market trends, customer behavior, or maintenance schedules. It’s often applied to business forecasting, personalized marketing strategies, and risk management.
- Descriptive AI: Analyzes past data to generate insights, trends, and summaries, often used for business intelligence, customer analytics, and reporting.
- Prescriptive AI: Recommends actions to optimize results in decision-making processes. Often used for supply chain optimization, healthcare, resource allocation, and demand forecasting.
Many of these categories are beginning to be combined into powerful tools, which we will look at later in this article.
First, let’s take a look at some general applications of AI and how it is being used in business today.
How is AI Generally Used in Business?
Across industries, AI’s applications are wide-ranging and increasingly accessible:
- Email Management: Tools prioritize critical messages or reduce clutter, improving productivity. I personally enjoy using SaneBox to help flag important emails and reduce inbox clutter, as it saves me a lot of time.
- Document Creation: AI helps draft workplace documents like job descriptions or agreements more efficiently. While generating legally binding documents without an attorney is not advisable, creating simple “handshake” agreements to document conversations and decisions can be highly beneficial for clarity and record-keeping.
- Marketing Automation: AI optimizes ad targeting, personalization, and campaign performance in real-time. It also enables greater quantity of reach outs, as you may have noticed by your LinkedIn inbox.
- Note-Taking: Tools like Fathom AI Notetaker help capture and organize meeting notes, enabling better collaboration and recall.
From time-saving tools to improved decision-making, these applications demonstrate AI’s value in streamlining day-to-day operations no matter the industry.
Cautions When Using AI
While AI is incredibly promising, it’s not risk-free. Manufacturers must consider several challenges:
- Security: AI systems need robust safeguards to prevent data breaches or cyber threats.
- Privacy: Training AI models with sensitive data can inadvertently expose confidential information to people outside the organization who are using any agent that uses the same AI model. Prioritize data protection to comply with privacy regulations.
- Confidentiality: Carefully manage user information to protect privacy. For example, if you use something internally that unintentionally exposes another company’s trade secrets to your team without them knowing you are bound by an NDA, it can put your organization at risk in ways you might not have anticipated.
- Bias: Models trained on incomplete or skewed datasets can develop biases that lead to flawed decisions. These biases include cultural biases but may also lead models to favor a particular decision or recommendation that may not be appropriate for your needs.
- Accuracy: AI occasionally misinterprets data or generates incorrect results. Organizations must recognize this and implement rigorous testing and validation procedures to address biases and errors.
- “Hallucinations”: Some AI tools may fabricate information altogether, which can lead to costly errors if left unchecked. An infamous example is the “strawberry” hallucination where the AI model is unable to correctly identify the number of ‘r’s in the word strawberry.
Despite its many benefits, rising popularity and constant improvement, AI is still relatively new and prone to mistakes. Exercise significant caution by protecting data, security, privacy, confidentiality, making sure you’ve adequately tested your AI products and ensure you have not introduced biases if you’re looking to use it for decision support. Strategic oversight and systematic testing are crucial when implementing AI to mitigate these risks and reap the benefits.
AI Adoption in Manufacturing
Manufacturers are ahead of the curve in AI adoption. A 2023 Machine Design survey revealed that 93% of respondents reported moderate functionality of AI in their operations. AI is already making significant inroads in predictive maintenance, demand planning, and even workforce automation.
That said, the U.S. remains slightly behind other regions in terms of AI deployment at scale. According to Datamation, 51% of European manufacturers implement AI, compared to 30% in Japan and only 28% in the U.S.
While reports on the overall impact on productivity varies, one study showed that organizations that successfully adopt AI often see up to 11.5% performance gains. This shows that organizations who are able to make significant AI implementation have enormous potential for competitive advantage.
Key Use Cases for AI in Manufacturing
Manufacturing is a key industry where AI is driving significant innovation with tangible benefits. These four areas are use cases I believe have the greatest possibility for return:
- Predictive Maintenance
AI analyzes sensor data to identify potential equipment issues, enabling timely maintenance and reducing costly downtime.
- Quality Control:
Machine Vision paired with AI algorithms can detect defects and ensure consistent product quality for every item—far more effective than traditional sampling methods.
- Demand and Supply Chain Planning:
AI analyzes global logistics trends (e.g., weather events affecting shipments) to improve inventory management and purchasing strategies.
Additionally, AI models have the capability to forecast market demand, enabling companies to optimize their inventory, identify seasonal market opportunities, and streamline production processes.
- Raw Material Price Forecasting:
Forecasting fluctuations in raw material prices based on global logistic trends can be really helpful in supporting strategic purchasing decisions and cost management.
These four use cases demonstrate some of the most impactful areas manufacturing can use to drive efficiency, quality, and cost savings when properly implementing AI in their business strategies.
The Difficult Reality of AI Implementation
Implementing AI in manufacturing is not without its hurdles. According to a recent McKinsey survey, 83% of companies believe AI has or will have noticeable impact, but only 27% are seeing current value from current AI projects. However, the general attitude remains optimistic, as 56% say they expect to see value in the next 2-5 years.
The reality remains bleak, however. In a recent Deloitte survey, 91% of AI projects fail to meet expectations.
Imagine for a moment that you are considering launching an AI project. Based on the projected ROI the project should pay for itself in two years. Now you find out that it is not meeting those expectations, and those 2 years could go out to five years, ten years, or even worse. The rate of unmet expectations is a real challenge and something that we encounter quite often.
By and large, the most daunting and significant challenge manufacturing companies face when implementing AI is the quality of their data.
Manufacturers generate vast amounts of data. 1,812 petabytes of data are generated annually. To put this into perspective, one movie on average is around 1 gigabyte, meaning 1,812 petabytes is equal to roughly 1.8 billion movies.
The quality and availability of data has a tremendous impact on the success of AI projects. Without high-quality data, AI models cannot function to their full potential and may produce inaccurate or biased results.
Incomplete or poorly organized datasets can weaken AI’s effectiveness. If companies want to ensure the success of their AI implementation, they must invest in data collection, data cleaning, and data management.
Preparing for AI in Manufacturing
To succeed with AI in manufacturing, preparation is key. Here’s what manufacturers should focus on in 2025 to be prepared to take full advantage of AI:
Transformation is not easy, but it doesn’t have to be impossible. Take control of your project’s success today and schedule a free 30-minute consultation to find out how Victoria Fide can equip you for transformational success.
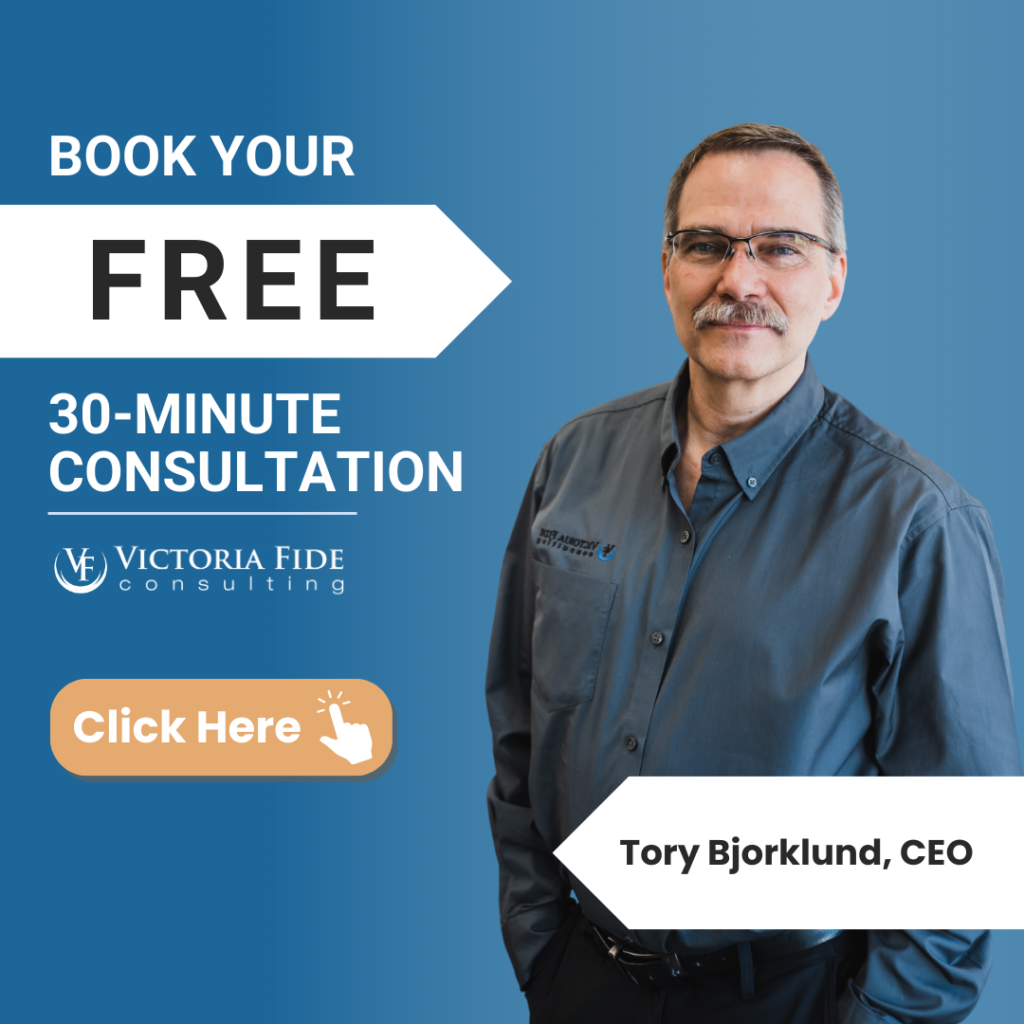
1. Build Data Maturity
Data maturity goes beyond data quality—it encompasses the structure, completeness, and collection methods of your data. To achieve true data maturity, companies must build a robust data infrastructure and adopt efficient collection processes. This includes organizing data to ensure completeness and maintaining rigorous data quality practices.
2. Automate Processes
Optimize workflows by automating tasks you intend to use with AI. For example, if you’re using spreadsheet-based production planning, you will not be able to implement AI in that process until after you’ve transitioned to an automated MRP system. Only once you have standardized data and processes will you be able to integrate AI to optimize those processes.
3. Start Small, Scale Fast
Begin with small internal pilot projects and scale up once AI’s benefits are clear. This will lower the risk for your organization both with costs and – as mentioned previously – privacy concerns.
For example, if you want an AI agent to help customers answer questions about products using data from your inventory system, you will want to make sure the data doesn’t include cost information unless you want your customers to have access to your cost information. Start with a small proof of concept and train it internally until you are confident it meets your requirements for security, privacy, and confidentiality.
4. Upskill Your Workforce
While AI is a powerful tool, it is useless without people to train it, prompt it, and vet its outputs. Companies must invest in their team’s skill development, focusing on AI literacy and problem-solving with AI tools.
Take prompts, for example. It takes some training to utilize tools like – chat systems effectively, largely in part due to the skill it takes to provide the right prompts. “Prompt Engineer” is already a job title that has cropped up to fill this need, and while I’m hesitant to say you need a specialist for this, it accurately reflects the need for training your workforce to be able to make effective use of the AI tools and systems.
One of the biggest concerns with AI is that it will replace human workers and unemployment rates will continue to rise. A survey done by KPMG in March 2024 confirmed this assumption when leadership said they anticipated AI leading to a net job loss. However, only a few months later, these same people changed their tune and said they believe they will need to hire more people in spite of implementing AI.
AI is not going to be replacing jobs; it will only be changing them. When companies invest in their workforce through training and providing support, employees will feel empowered, not replaced, by the technology, allowing companies to scale with AI rather than substitute.
5. Manage Organizational Change
You must foster a culture of experimentation, innovation, and collaboration while addressing fears about job displacement. Aim to create an environment where employees actively seek opportunities to harness AI for growth and efficiency.
Ethical considerations are also an important part of navigating AI adoption, particularly with generative AI tools that may pull information from other sources without properly attributing those sources. It’s crucial to educate your employees on the ethical uses of AI and create an environment that is conducive to having conversations around these concerns.
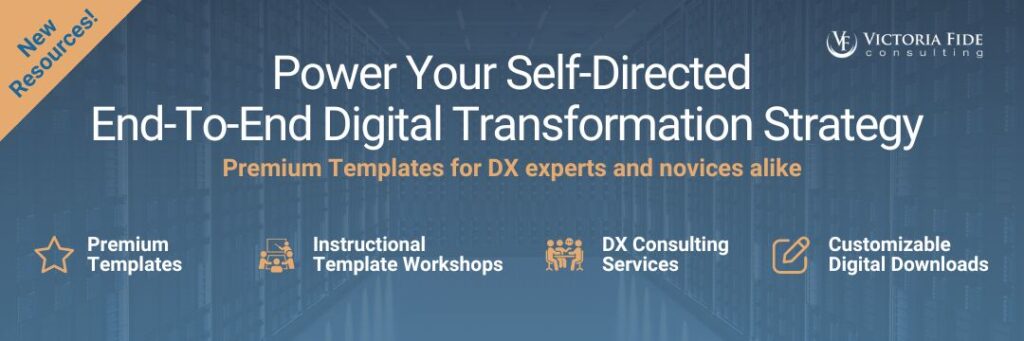
Why AI is Essential for Manufacturing’s Future
The anticipated potential for AI is high and growing exponentially. By automating routine tasks, optimizing workflows, and uncovering actionable insights from data, AI empowers businesses to enhance productivity and maintain a competitive edge in an increasingly globalized market.
However, implementing AI is not without its challenges, as more than 90% of AI projects fail to meet expectations. If they want to integrate AI in their processes, companies must prioritize data maturity, process automation, and workforce training.
Businesses have the opportunity to achieve significant scalability and efficiency gains, and the key lies in creating a foundation that will balance technological advancement with human adaptation, creating a future where AI and people work seamlessly together.